How To Paint Wainscoting: A Step-By-Step Guide
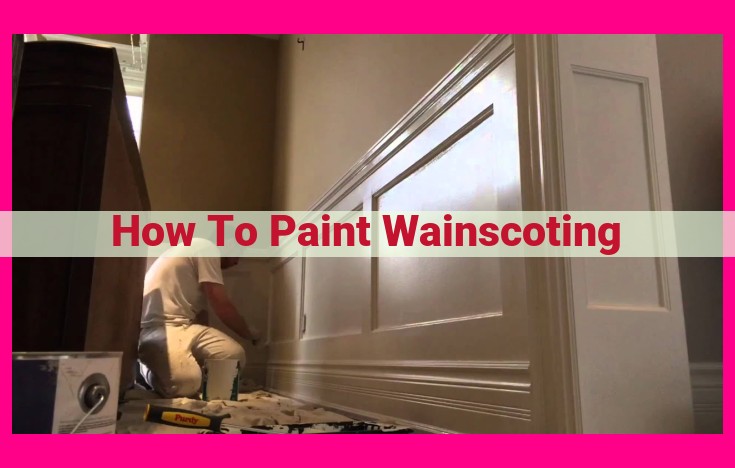
To paint wainscoting, gather materials including primer, paint, brushes, and drop cloths. Remove switchplates and covers, and clean the wainscoting surface. Apply a coat of primer and let dry. Use a brush to paint the vertical stiles and rails, then roll the paint onto the panels. Let dry and apply a second coat of paint. Replace switchplates and covers after the paint dries.
Understanding the Cornerstones of Closeness Score Computation
In the realm of network analysis, the concept of closeness score plays a pivotal role in unraveling the intrinsic relationships and connections within complex systems. It serves as a measure of how proximate a node is to all other nodes in a network, providing invaluable insights into the node’s position and influence.
The essence of a closeness score lies in its constituent elements, which form the bedrock upon which this metric is constructed. Three fundamental elements stand out as the cornerstones of closeness score computation:
-
Distance: This element captures the physical or abstract separation between nodes. It can manifest in various forms, such as geographical distance, temporal distance (e.g., time difference), or even social distance (e.g., perceived difference in status or familiarity).
-
Time: Time plays a crucial role in quantifying closeness, particularly in dynamic networks where relationships evolve over time. By measuring the temporal proximity between nodes, closeness score can reveal how quickly or easily information, resources, or influence can flow through the network.
-
Relationship strength: The strength of a connection between nodes is a critical factor that modulates the closeness score. This strength can be quantified through parameters such as frequency of interaction, intensity of the relationship, or the perceived trust between the connected nodes. By incorporating relationship strength, closeness score can differentiate between mere acquaintances and close-knit relationships, revealing the network’s underlying hierarchical structure.
These elements gracefully intertwine to form the foundation of closeness score computation. By judiciously combining distance, time, and relationship strength, network analysts can unveil the intricacies of complex systems, unraveling patterns of connectivity and identifying influential nodes.
Explain how these components influence the overall computation of the closeness score.
Influence of Components on Closeness Score Calculation
The closeness score is a metric used to measure the proximity between two or more entities. It is calculated using various components, such as distance, time, and relationship strength. Understanding how these components contribute to the overall computation of the closeness score is crucial for accurate interpretation.
Distance: Distance is a fundamental factor influencing the closeness score. In general, entities that are geographically closer tend to have a higher closeness score. The distance metric used can vary, such as Euclidean distance or network distance, depending on the nature of the data.
Time: Time plays a role in calculating the closeness score when it comes to temporal relationships. For example, in social network analysis, the time since the last interaction between two individuals can affect their closeness score. Entities that have interacted more recently tend to have a higher closeness score.
Relationship Strength: Relationship strength refers to the intensity or weight of the connection between two entities. This component is particularly important in weighted closeness scores, where the weight of each relationship is considered. The weight can be assigned based on factors such as frequency of interaction, intimacy, or trust. Entities with stronger relationships will contribute more significantly to the overall closeness score.
By combining these components, the closeness score provides a comprehensive measure of proximity that takes into account not only geographical closeness but also temporal and relational aspects. It helps identify the most closely related entities within a given dataset, which can be valuable for various applications such as community detection, link prediction, and network optimization.
The Intriguing World of Closeness Scores: Unveiling Different Styles
In the captivating realm of network analysis, the concept of closeness score holds immense significance. It measures the extent to which actors within a network are connected, revealing their level of influence and reach. Among the diverse styles of closeness scores, the weighted and unweighted variations stand out, each with its own unique advantages and drawbacks.
Unveiling the Simplicity of Unweighted Closeness Scores
Unweighted closeness scores, like unadorned diamonds, possess a straightforward elegance. They treat all edges in the network equally, assigning them the same weight or value. This simplistic approach renders the computation process relatively swift and effortless. However, its impartiality can be an Achilles’ heel, failing to capture the nuanced importance of specific connections.
Exploring the Nuances of Weighted Closeness Scores
Weighted closeness scores, on the other hand, delve deeper into the intricate tapestry of network connections. They acknowledge that not all edges are created equal, assigning weights based on their strength or significance. This intricate weighting system allows for a more refined and realistic representation of the network’s dynamics. Yet, the added complexity can introduce challenges in interpreting the results.
Navigating the Advantages of Unweighted and Weighted Styles
Unweighted closeness scores shine when the focus is on overall connectivity, providing an accessible measure of nodal centrality. Their simplicity makes them ideal for quick assessments of network structure. Weighted closeness scores, however, offer a more comprehensive understanding of influence and proximity within the network. They excel in scenarios where certain connections hold greater value than others.
Choosing the Perfect Style for Your Network Adventure
The choice between unweighted and weighted closeness scores hinges on the specific research question and the nature of the network under investigation. For a comprehensive overview of the network’s connectivity, unweighted scores suffice. However, if the aim is to pinpoint the most influential nodes or uncover the hidden power structures within the network, weighted scores are the wiser choice.
Embarking on the Closeness Score Journey
Whether you choose the unadorned simplicity of unweighted scores or the nuanced elegance of weighted scores, the journey of exploring closeness measures is a captivating one. By unraveling the complexities of network connections, you’ll gain invaluable insights into the dynamics that shape the world around you.
The Impact of Style Selection on Closeness Score Interpretation
The Tale of Two Scores
In the realm of network analysis, the closeness score is a measure that quantifies how well-connected a node is to other nodes in a network. While the formula for calculating the closeness score may seem straightforward, the choice of style can have a profound impact on its interpretation.
Imagine two nodes, Node A and Node B, in a network. Both nodes have the same number of connections and the same average distance to other nodes. However, if Node A’s connections are more uniformly distributed than Node B’s, the choice of style can lead to different closeness scores.
Weighted vs. Unweighted Styles: The Case of Uniformity
In a weighted style, each connection is assigned a weight based on its strength. In our example, since Node A has more uniform connections, each of its connections will receive a lower weight. This results in a lower overall closeness score for Node A compared to Node B.
Conversely, in an unweighted style, all connections are treated equally. In this case, Node A’s uniform connections will not affect its closeness score, which will be the same as Node B’s.
Selecting the Appropriate Style for Your Needs
The choice of closeness score style depends on the specific application and the desired interpretation. If you want to emphasize the connectivity of a node to its nearest neighbors, an unweighted style may be more appropriate. However, if you want to highlight the influence of strong relationships, a weighted style is more suitable.
Understanding the impact of style selection on closeness score interpretation is crucial for using this measure effectively in network analysis. By carefully considering the characteristics of your network and the intended interpretation of the results, you can select the style that best aligns with your research goals and provides meaningful insights.
Calculating Closeness Score: A Comprehensive Guide to Techniques
Understanding the intricacies of calculating a closeness score is essential for accurately assessing the proximity of nodes within a network. Various techniques have been developed to compute this vital metric, each with its own strengths and limitations. Here’s a comprehensive guide to the most widely used techniques:
1. Weighted Centrality
Weighted centrality assigns different weights to connections based on their strength or importance. Nodes with stronger connections are given higher weights, resulting in a more accurate representation of their influence within the network. This technique captures the true closeness of nodes by considering the quality of their connections, not just their quantity.
2. Unweighted Centrality
Unweighted centrality treats all connections equally, regardless of their strength. This technique is straightforward to compute and provides a quick estimate of closeness that can be useful in exploratory analyses. However, it may overemphasize the quantity of connections and underestimate the influence of nodes with stronger relationships.
3. Harmonic Centrality
Harmonic centrality takes into account the shortest paths between nodes. It assigns a closeness score to a node based on the average of the harmonic mean of all shortest paths to other nodes in the network. This technique provides a balanced measure of closeness, considering both the number of connections and the distances between nodes.
4. Radiality
Radiality calculates closeness by measuring the sum of the distances between a node and all other nodes in the network. Nodes with a smaller sum of distances are considered closer to the center of the network and have higher closeness scores. This technique emphasizes the geographic proximity of nodes and is often used in spatial analysis.
5. Eigenvector Centrality
Eigenvector centrality assigns scores to nodes based on the influence of their neighbors. Nodes connected to highly influential nodes receive higher scores, which creates a cascading effect throughout the network. This technique captures the global importance of nodes and is useful for identifying key individuals or entities within a network.
Understanding the different techniques used to calculate closeness scores is crucial for selecting the appropriate method for your specific application. Consider the nature of your data, the goals of your analysis, and the computational resources available to make an informed choice.
Techniques for Calculating Closeness Score
In the realm of network analysis, calculating the closeness score is a crucial step in understanding the connectedness and influence of nodes within a network. This score measures how close each node is to all other nodes, providing insights into the network’s overall structure and functionality.
Several techniques exist for computing the closeness score, each utilizing distinct mathematical formulas and algorithms. These techniques vary in their complexity, accuracy, and applicability to different types of networks.
Distance-Based Techniques
One common technique measures closeness by calculating the average distance between a node and all other nodes in the network. The distance between two nodes can be defined as the number of edges connecting them. Nodes with shorter average distances to others are considered closer and thus have higher closeness scores.
Time-Based Techniques
Another approach considers the time it takes for information or influence to spread from one node to another. This technique assigns weights to edges based on the time delay associated with traversing them. The closeness score of a node is then calculated as the average weighted time it takes for information to reach all other nodes from that node.
Weighted Closeness Techniques
In some cases, it may be necessary to consider the strength of relationships between nodes. Weighted closeness techniques assign weights to edges based on the importance or strength of the relationship they represent. The closeness score is then computed as the average weighted distance between a node and all other nodes, taking into account the strength of the relationships.
By understanding the different techniques used to calculate closeness score, researchers and analysts can select the most appropriate method for their specific network analysis applications. Each technique offers unique advantages and limitations, and the choice depends on factors such as the type of data available, the network’s characteristics, and the desired level of accuracy and detail required.
Calculating Closeness Scores: A Comprehensive Guide to Techniques, Tools, and Materials
Understanding the closeness score is essential for analyzing and interpreting social networks. In this comprehensive guide, we’ll delve into its intricacies, highlighting the distinct techniques, tools, and materials involved in its computation.
Diving into Techniques: Strengths and Limitations
Symmetric vs. Asymmetric Techniques:
- Symmetric techniques measure closeness in both directions, providing a balanced perspective of the relationships.
- Asymmetric techniques consider only one direction, which can be useful in directed networks, such as social media platforms where user interactions are often one-sided.
Distance-Based Techniques:
- These techniques quantify closeness based on the physical or virtual distance between nodes.
- They are straightforward and intuitive but may not fully capture the complexities of social relationships.
Time-Based Techniques:
- These techniques consider the time elapsed between interactions to determine closeness.
- They are particularly suitable for studying dynamic networks where relationships evolve over time.
Weighted vs. Unweighted Techniques:
- Weighted techniques assign different weights to different types of relationships, reflecting their perceived importance.
- Unweighted techniques treat all relationships equally, which can lead to biased scores in networks with varying relationship strengths.
Navigating Tools and Materials
Choosing the right tools and materials is crucial for accurate closeness score calculations.
Software Tools and Libraries:
- NetworkX and igraph are popular Python libraries for network analysis and closeness score computation.
- Pajek and UCINET offer specialized software for social network analysis, including closeness score calculation.
Data and Materials:
- The type of data used, such as adjacency matrix or edge list, determines the choice of technique and tool.
- Data quality and preprocessing are essential for ensuring reliable results.
- Gathering and preparing the necessary materials, such as relationship data, spatial coordinates, or time stamps, is a crucial step in the process.
List and describe the software tools and programming libraries available for computing the closeness score.
Tools for Unveiling Closeness: Software Solutions and Programming Libraries
Unveiling the intricacies of closeness requires robust computational tools. Software engineers and data scientists have crafted an array of software tools and programming libraries specifically designed to calculate this elusive metric.
Among the most popular software tools is the Gephi platform, renowned for its user-friendly interface and extensive customization options. Its intuitive design allows researchers to visualize and analyze complex network data, making it an ideal choice for exploring closeness dynamics.
For those seeking a more programmatic approach, several programming libraries offer powerful capabilities for computing closeness scores. The NetworkX library in Python provides a comprehensive set of functions for manipulating and analyzing network structures, including the calculation of closeness centrality.
Another notable library is igraph in R, which offers a versatile toolkit for graph analysis and visualization. Its specialized algorithms efficiently handle large-scale network data, making it suitable for complex scenarios.
When selecting the right tool or library, consider the size and complexity of your network data. For smaller networks, user-friendly software tools may suffice. However, as networks grow in size and complexity, the power and flexibility of programming libraries become essential.
Regardless of your choice, these tools and libraries empower researchers with the means to delve into the intricate web of closeness, unraveling the hidden connections and patterns that shape our social and technological landscapes.
Provide instructions on how to use these tools and select the most appropriate tool for specific applications.
Optimizing Closeness Score Calculations for Your Application
In our previous sections, we’ve explored the essential components, styles, and techniques involved in calculating closeness scores. Now, let’s dive into the practicalities of applying this knowledge to your specific use cases.
Selecting the Right Tool for the Job
Choosing the appropriate software tool or programming library is crucial for efficient and accurate closeness score calculations. Each tool offers its own set of features and capabilities, tailored to varying application requirements.
For large-scale data analysis: Opt for tools like Hadoop or Spark, known for their robust big data processing abilities.
For real-time computations: Consider streaming platforms such as Apache Kafka or Storm, designed to handle high-velocity data streams.
For network analysis: Explore specialized libraries like NetworkX in Python or igraph in R, which offer advanced algorithms tailored for network topology analysis.
When selecting a tool, carefully consider the following factors:
- Data size and complexity: Choose a tool that can handle the volume and complexity of your data efficiently.
- Computation time: Determine the acceptable trade-off between accuracy and computation time, and select a tool that meets your performance requirements.
- User-friendliness: Opt for tools with intuitive interfaces and documentation, especially if you’re not technically proficient.
- Integration with existing systems: Ensure that the chosen tool can easily integrate with your existing data infrastructure and workflows.
By following these guidelines, you can select the most appropriate tool for computing closeness scores in your specific application scenario, ensuring accurate and efficient results that enhance your decision-making capabilities.
The Importance of Data and Materials in Closeness Score Calculation
In the realm of network analysis, calculating closeness scores plays a crucial role in understanding the connectedness and proximity of individuals or entities within a network. To ensure accurate and meaningful results, the quality and preparation of the data and materials used are paramount.
Understanding the Data Requirements
The types of data required for closeness score calculation depend on the specific application and the network being analyzed. However, some common types of data include:
- Node Data: This includes information about individual nodes in the network, such as their identification, attributes, and relationships with other nodes.
- Edge Data: This data represents the connections between nodes and can include information such as distance, weight, and directionality.
Data Preprocessing for Accuracy
Before calculating closeness scores, it’s essential to preprocess the data to clean, transform, and standardize it. This process ensures data consistency and accuracy, which directly impacts the reliability of the calculated scores. Some common data preprocessing techniques include:
- Missing Value Imputation: This involves identifying and filling in missing values in the data using appropriate methods, such as mean or median imputation.
- Outlier Detection and Treatment: Removing or adjusting extreme values that can skew the results is crucial.
- Data Normalization: This technique scales the data to a consistent range, improving comparability and reducing the impact of outliers.
Guidelines for Gathering and Preparing Data
To collect and prepare data effectively for closeness score calculation, consider the following guidelines:
- Identify the relevant data sources: Determine where the necessary data can be obtained, such as surveys, databases, or social media platforms.
- Establish data collection protocols: Define clear procedures for gathering data to ensure consistency and reliability.
- Use appropriate tools and techniques: Leverage data management tools and statistical software to efficiently process and prepare the data.
- Document the data preparation process: Maintain a detailed record of the data preparation steps performed for transparency and reproducibility.
By carefully selecting, preparing, and preprocessing the data and materials, researchers and analysts can ensure the accuracy and reliability of their closeness score calculations, leading to meaningful insights into network structures and relationships.
Materials: The Foundation for Precise Closeness Scores
The Importance of Data Quality and Preprocessing for Accurate Results
Just like building a sturdy house requires high-quality materials, calculating an accurate closeness score demands pristine data. Impurities and inconsistencies in your data can distort the score, leading to misleading conclusions. Data preprocessing plays a crucial role in ensuring the integrity of your findings.
Think of it this way: imagine you’re trying to measure the distance between two cities using a map. If the map contains errors, your measurements will be off. Similarly, if your data contains missing values, outliers, or duplicate entries, your closeness score will be unreliable.
Preprocessing: Refining Your Data for Success
Data preprocessing involves a series of steps to cleanse and prepare your data for analysis. These steps may include:
- Eliminating missing values: Impute missing values using statistical methods or by referring to external data sources.
- Removing outliers: Identify and remove extreme values that can skew the score.
- Handling duplicate entries: Remove duplicate data points to prevent overcounting and ensure accuracy.
- Standardizing data: Convert different data formats and units to a common scale for easier comparison.
By meticulously following these preprocessing steps, you can enhance the quality of your data and ensure that your closeness score accurately reflects the underlying relationships between individuals or entities.
Gathering and Preparing the Necessary Data
The type of data you need for calculating the closeness score depends on the specific application. Common data types include:
- Spatial data: Coordinates or distances between locations.
- Temporal data: Time stamps or durations.
- Social network data: Connections and relationships between individuals.
It’s essential to collect complete and accurate data from reliable sources. Carefully plan your data gathering strategy and consider using data validation tools to ensure the integrity of your data.
Calculating Closeness Scores: A Comprehensive Guide
Understanding the closeness score is essential for analyzing the relationships between individuals or objects. This score quantifies the proximity and strength of connections, making it a valuable tool in various fields. This guide will provide a comprehensive overview of the components, styles, techniques, and tools involved in calculating closeness scores.
Components of a Closeness Score
- Distance: This measures the physical or metaphorical distance between entities.
- Time: Considers the duration of relationships or interactions.
- Relationship Strength: Assesses the intensity and quality of connections.
These components are combined to create a numerical value that represents the closeness between entities.
Styles of Closeness Scores
- Weighted: Assigns different weights to components based on their importance.
- Unweighted: Treats all components equally.
The choice of style depends on the specific application. Weighted scores emphasize crucial components, while unweighted scores provide a more balanced view.
Techniques for Calculating Closeness Scores
Various techniques exist to calculate closeness scores, including:
- Geodesic Distance: Calculates the shortest path between nodes in a network.
- Euclidean Distance: Measures the straight-line distance between entities in a coordinate system.
- Temporal Closeness: Focuses on the frequency and timing of interactions.
- Weighted Closeness: Assigns weights to components, such as relationship duration or emotional intensity.
Tools for Computing Closeness Scores
Several software tools and programming libraries facilitate the computation of closeness scores:
- Gephi: A free and open-source network analysis software.
- NetworkX: A Python library for analyzing complex networks.
- SciPy: A Python library offering advanced statistical and numerical functions.
Selecting the appropriate tool depends on the size and complexity of the data, as well as the specific analysis requirements.
Gathering and Preparing Materials
Accurate closeness scores require reliable data.
- Data Types: Collect data on distance, time, and relationship strength, ensuring its completeness and accuracy.
- Preprocessing: Clean and transform the data to ensure compatibility with the chosen tools and techniques.
- Data Quality: Verify the data’s consistency and reliability by performing checks for missing values and outliers.
By following these guidelines, you can gather and prepare the materials necessary for calculating valid and meaningful closeness scores.